The Snapdragon 8 Gen 3, Qualcomm’s new high-end mobile chip, ushers in the arrival of generative AI running locally, on a smartphone, without going through the cloud. With the promise of better protection of private data.
The launch of the new flagship chip for 2024 smartphones at the end of October 2023 by Qualcomm, the Snapdragon 8 Gen 3, is an opportunity to see the arrival of a new element in the narrative of the general public uses of AI: the protection of privacy. If, in the past, most presentations of high-end chips – Apple included – consisted of announcing more power, graphics or otherwise, or promising wonders in autonomy, the Snapdragon 8 Gen 3 has other arguments in no longer in his suitcase. Which all starts with a performance indicator: capable of executing some 45 TOPS according to Qualcomm – or 45,000 billion AI operations per second – this cutting-edge chip takes advantage of this deluge of combined power (read further) to achieve a small miracle: being able to run complex AI models locally, that is to say on a device, without external help. A small revolution which promises two major advantages: a reduction in latency and energy consumption on the server side, and better protection of the private data of private users, the local execution of AI avoiding having to run our personal data by Internet giants. At least in theory…
Generative AI: the problem of personal data
You have heard of these models, particularly with the generative AI fever which hit the world at the start of 2023: ChatGPT, MidJourney, DALL-E and other Bard. Programs capable of generating entire sections of code, writing a cover letter structure or creating a Vermeer-style table with a few key words. In the furnace of supercomputers loaded with GPUs, powerful models were born and invaded the cloud… Bringing with them many questions, all legitimate. The first to emerge and start flooding the courts are copyright issues, with OpenAI-like giants apparently training their AIs on copyright-protected files – something artists still in exercise, obviously did not miss it… Nor appreciated it!
But for the general public, another problem arises: what do the servers do with our requests? Do they record parts of our lives, commercial or confidential information, when we ask them to generate a text or an image? And these personal assistants, well nestled in the cloud and who comb our calendars and our emails to prepare alerts for us, what do they do with our data too? Are they hackable?
Faced with these risks and flaws, a double mechanism is being put in place: the emergence of less complex models on the one hand, and the rise in power of AI calculations on the chip side. Two trends which allow the arrival of a new wave of generative AI capable of working not in the fog of the cloud, but in the palm of our hand. But as we will see, greatness of soul is not the only driving force behind the industrialists’ approach…
Artificial intelligence: enormous computing needs
In the past, the NPU (Neural Processing Unit in English, or neural network acceleration chip) was presented as the only artificial intelligence chip. This was never completely the case – AI calculations are distributed across the CPU, GPU or NPU depending on their nature – but it became completely false in 2023. Now other chips like the modem, the processor image processor or the processor in charge of the sensors have their own AI calculation units (tensors or others).
In the case of the maximum AI power of 45 TOPS announced by Qualcomm for the Snapdragon 8 Gen 3, it must be understood that this is a combined power of both the CPU, the GPU and the NPU. In addition to their different natures – noise reduction in communication, blurring, etc. –, AI algorithms are increasingly complex and can use several types of computing processors during their execution.
If the coupling of these chips makes it possible to develop more overall power, another element allows the execution of such large and efficient models: the simplification of part of the calculations. In the past, AI calculations had to be very precise and were performed on integers of great complexity. Over time, engineers and other developers have managed to design models using lower levels of precision by training AI to improve the calculations. a posteriori. The latest trend has made it possible to reduce this level of precision to Int4 (4 bits), instead of 8 or even 16 bits.
If this is a detail for you, it is a panacea for processors, which see calculation times reduced exponentially when this precision decreases. Coupled with permanent increases in processor power, this will allow, at the end of 2023, a manufacturer like Qualcomm to launch a chip generating less than 5 W to support complex models with more than 10 billion parameters. Or how to put the power of a ChatGPT (light) in the palm of your hand!
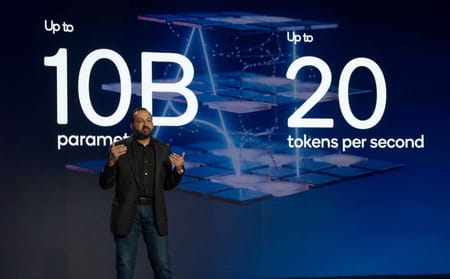
Local generative AI: simpler and more customizable models
The primary benefit of running AI models in smartphones is latency. Often overwhelmed with requests, ChatGPT, MidJourney and other Dall-Es impose queues, limits on the number of requests, etc. While promoting subscriptions to make their servers profitable. If the models supported by personal terminals – PC included – are more simplified, they already have the advantage of not depending on a network chain or an order of priority. Thus, Qualcomm promises generation of images in the space of less than a second when it sometimes takes several dozen when typing in a remote server as we do with online AI.
The other benefit, less visible and yet more critical, is that of better protection of privacy. If, for the moment, no scandal has tainted OpenAI and others, the fact remains that the amount of potentially sensitive data that has passed through their servers is enormous. From ChatGPT to Microsoft Copilot, all these AIs run on powerful remote computers in the cloud. But, as the expression goes, “There is no cloud: it’s just someone else’s computer.” And if automation has greatly amused the general public, it has also and above all excited professionals, with some companies even rushing to subscribe to Chat-GPT combined with the recruitment of data entry engineers capable of quickly developing instructions. (prompt) relevant and effective (the prompt engineers), and thus get rid of certain highly automatable positions…
However, sending a request like “draw a cat that looks like James Bond lost in the tundra in the style of Piero della Francesca”, it is different for sending customer data to the cloud to generate an Excel table. Or let an AI “graze” your personal data to improve your personal assistant.
This is where systems like LLaMA (the linguistic model of Meta, the parent company of Facebook and Instagram in particular) changes the situation. This lightweight LLM allows the design of models that are easier to execute by small machines. By reducing the weight of models – either by reducing their precision or by specializing them – the industry is in the process of producing AI that can now be executed on mobile chips. This greater lightness coupled with the possibility of local execution should promote the emergence of more customizable and secure AI models. Because the additional protection of privacy that this implies makes it possible to mill, without external supervision, feeding small AIs browsing your calendar, your emails, your photos to generate actions – suggestions, email reminders, etc. Besides the fact that these local AIs will never eclipse AIs in the cloud – a supercomputer has “slightly” more power than your smartphone! –, this development is not only the honorable amends of an industry which would like to atone for having siphoned off your data. It’s also a question of…electric bill.
Local AI: limit the energy bill
The tech industry is pretty Cartesian. And, like you, she has to pay her electricity bills every month. However, energy prices have been on quite a roller coaster ride over the past two years. And any reduction in the bill is welcome: why run an Intel Xeon processor core or a large Nvidia GPU to generate two paragraphs of text on a server when this task can be executed on a low-power chip like SoCs ( system on a chip, all-in-one chip) of smartphones?
You will note that we used the term “limit” and not “reduce”. Contrary to the dreams of blissful technophiles, the transfer of part of the calculations from the cloud (and therefore from the servers) to the “edge”, that is to say the terminals at the end of the line, should not reduce electricity bills , but slow down their increase. On the one hand, the most advanced AI will always be executed in the cloud, as much for reasons of computing power as for reasons of memory – size of models, etc. Then, this deviation only concerns the execution of established models. However, the race between companies in the segment also hinges on complexity and therefore on the training of AI.
But here’s the thing: even to produce a lightweight model, it takes days of calculations. And the ChatGPT earthquake resulted in an acceleration of R&D in the field of generative AI. Leading to two waves: the explosion in the development of specialized models on the one hand, and the rise in power of super AI called foundation models. Bigger, more global and requiring even more training time. It is this training that is singled out by some, because it requires more and more computing time with increasingly powerful chips. Thus, Nvidia’s latest GraceHopper platform is a large server blade displaying no less than 1000 W on the meter. And Nvidia has even developed a supercomputer combining the power of 256 units of this monster (we’ll let you calculate the necessary electricity consumption…)
Now that the Snapdragon 8 Gen 3-style superchips are soon here, all that remains is for the smartphone software ecosystem to develop and integrate these models developed with huge megawatts. And hope that the uses are worth the effort!